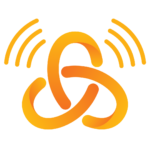
Description
Lily and David discuss the importance of working with multi-level data. The conversation highlights the need for integrating the concept of multi-level data into data literacy education, from basic to advanced levels, to help people better analyze and interpret complex data sets. They also touch on the practical implications of ignoring multi-level data, such as in the 2020 UK examination algorithm controversy, and consider the relevance to AI.
[00:00:00] Lily: Hello, and welcome to the IDEMS podcast. I’m Lily Clements, a data scientist, and I’m here with David Stern, a founding director of IDEMS.
Hi, David.
[00:00:14] David: Hi Lily, really nice to chat. We’re talking about something close to my heart today, multi level data.
[00:00:20] Lily: Absolutely, and I suppose we could start off by talking about, you know, this is the Responsible AI set of podcasts, where does this link in with Responsible AI?
[00:00:30] David: It’s not just about responsible AI that multi level data is important, but it is included there. It is one of the things where almost all data that people work with nowadays is inherently multi level. And yet still our analytic tools, by and large, aren’t set up to deal with multi level data.
We still tend to think about data skills of analysing data once it’s at a single level.
[00:00:58] Lily: So let’s jump in and say what we mean by multi level data.
[00:01:03] David: Absolutely, and I think, let’s forget about the AI complications and just think about data very simply. People tend to think about data and a good way to think about data is a rectangle. You have a rectangle of data and you know each column is a sort of variable, it has meaning and each row is an entry which has those properties with respect to the columns. So you have your, if you want your units which are your rows and you have your columns which are the properties of those units. Does that make sense? Can you clarify that for the audience?
[00:01:39] Lily: Yes. If we just think of a simple data frame or a simple spreadsheet if you think of your Excel spreadsheet, let’s say, which you may not like me using that example, but we will, you just have this flat rectangle of data and you have rows and you have columns.
[00:01:55] David: Yes.
[00:01:55] Lily: Now, the difference is, and why I said you may not like the using the Excel example, is because in Excel you can have multiple rows as a header.
[00:02:05] David: And why might you want that? You might want that because a whole set of columns are grouped together as being some sort of properties. If your person is your row then you might want to have all their physical attributes together as part of the same thing and you’d have height, weight and all sorts of things as their physical attributes. So you’d group together the physical attributes and so on. That would be an example.
[00:02:30] Lily: Or another one that I’ve seen is with farming data, where you might have something on cows, and all the cows attributes, and then sheep, and then all the sheep attributes, and then that’s kind of what we mean by multi level data.
[00:02:43] David: And that’s a really interesting one that you’re saying that the row there is let’s say a farm and within the farm you have cows and sheep. And so you have your cows’ columns and then you have your sheep’s columns and you know they could have some of the same types of columns so the number which would be the number of cows and the other one would be the number of sheep and so this would be a way of organizing the data.
Yeah. And so multilevel data is very common and out there, if we just look at a school, if we’re looking at a region and a bunch of schools, then we have different levels there. You have different schools as one level, and then within the school, you have the students or the pupils at a different level.
[00:03:23] David: And the classes they’re in, because of course all the students who are in a particular class probably have the same teachers, so it’s sensible to have some data which is related to the individual student. Another data might relate to the individual classes, in which case you would associate the teachers to the class, not the teachers to the students, and then another level might be the school level and all the school, everyone in the school is in the same location. So if you have the geolocation, that’s a school level data. It doesn’t need to be at the individual level and so on.
[00:03:55] Lily: You might want to compare the kind of individuals to one another within a class, you might want to compare teachers, it might be across lots of different schools. So in the UK, we sit exams called SATS in kind of year two and year six. So when you’re about seven, eight, and around 11 years old, and it might be that you want to compare how the students performed with their teacher. So you want to look at all of those year six pupils across different schools.
[00:04:23] David: But if you’re doing it at the teacher level, then you’re doing a summary of the students to the teacher. And so this is the thing, you have all these tools which enable you to move from one level to another. All this is relatively natural stuff to do when you work with data.
So we’ve got an idea, maybe I should just come back to your farm example for a second, because I think this is a really interesting one. So your farm example, where you were looking at the farm level data, where you had certain sets of columns, which are the cow columns, and then another set of columns, which are the sheep columns. One way of looking at that is that data doesn’t belong, the cow data doesn’t belong at the farm level. It probably belongs at the cow level. So maybe you want to have sort of cow level data where you could actually have information on the individual cows, which would then be summarized up so the number of cows would just be the summary of this.
So if you think about this, and this is when you’re thinking about the society we live in, more and more, we live in a society where actually you can get this micro level data. People are putting sort of clips in cow’s ears, which actually are intelligent clips, which you then transmit information, store information, so you’re getting cow level data.
And so inherently, we’re in a data rich world where there’s data coming out all over the place from remote sensing, from chips, from, smartphones, from all sorts of things in all sorts of different ways. And so we’re in this extremely data rich world. And quite often what happens is we then put all that data into something, but we sometimes forget the structure. If you’re doing this and you think about this in terms of a database. Before, this was when you had that complex data, it was all about being a database, putting it in a database. But, right now, we think of this even as unstructured data, having an unstructured database. Because we don’t necessarily know the structure of the data in advance.
And, we might want to bring together data, which is coming from different structures. We’re living in such a data rich world that there’s data coming in from all sorts of different angles. And yet in education, in all sorts of areas where people are taught how to work with data, they are not taught inherently about how to work with multi level data and the importance of multi level data. And even our tools, our analytic tools, they’re not really designed for multi level data.
And this is something where the importance of multilevel data is not for just people who are statisticians and automatically think, oh, that’s advanced because we need to do multilevel modelling, and since you’re doing multilevel modelling, you’re worrying about error at different levels. What does that mean? That means that you might have variability at the individual level and you might have variability, let’s say, at the class level, which is due to the difference in teachers, or the school level, which is due to the difference in the environment in the school. That would be the nature of multi level modelling.
[00:07:21] Lily: Sorry, and by variability we mean those differences that you see. Why is everyone not getting 80 percent on this test, someone gets 70, someone gets 90, you’ve got that variability there.
[00:07:31] David: Exactly, and variability is natural. And some of the variability is due to the differences between the students. Some of it is due to the differences between the teachers. Some of it is due to the differences between the schools. Being able to distinguish between what the source of that variability is, because you might not be able to do anything about the variability between the students in certain ways without a huge amount of extra finance and resources.
You know, the variability between the teachers, again, this is natural to some extent, but you might be able to find outliers who are particularly good, which you could learn from, or particularly bad, who you could help and support. And at the school level, you could potentially find opportunities to actually find where you could invest in improving the environment in particular ways which would be most cost effective.
Understanding the source of variability is really important and really valuable. And I’m not saying that people don’t do those analyses. What I am saying is that those analyses that people do to get these things at a different level, these are still considered advanced analyses. They shouldn’t be. Everybody should be thinking about this, about multilevel data. We shouldn’t be seeing this as an advanced topic. We should be seeing this as basic data literacy. Because it doesn’t need to be hard, it doesn’t need to be about having to use these very complicated multi level models.
No, it’s about looking at data differently, it’s about observing data, being able to visualize data let’s say, and interpret data with an awareness of variability at multiple levels, of the fact that data intrinsically has multiple levels and where is it coming from and what does it mean. This is something which I believe should be part of our basic data literacy, and yet it really isn’t. Very few people do this.
[00:09:23] Lily: Yeah, I didn’t do it in my bachelor’s or in my master’s, which were both in statistics.
[00:09:28] David: Yes, this is the thing. And if you think about that, that’s just insane, that you were a specialist, and yet, multilevel was still considered, no, that’s an advanced topic. Whereas it’s every single data set in the real world, pretty much. This is insane. And what are we actually teaching? Now, okay, people might say, oh, but that’s statistics. Statistics is over functioning. Data science is just as bad. In many different ways, this prioritization of multi level, data scientists haven’t embraced it as I would have hoped that they might.
Now, I’m not saying that statisticians or data scientists are bad at this. What I am saying is in thinking about data literacy and getting out data literacy concepts at scale, multi level is not included and it should be. That’s the key, if people are taking something away from this, that’s what I’d love them to take away.
I don’t often have something quite as militant that I want to get out of a podcast, but I would love it if data literacy really included these ideas about thinking about the level of your data, in really powerful ways and what the structures in your data should be. But we would need to think about and build tools differently to do so.
[00:10:46] Lily: And you mentioned one reason why it’s important which is that, you can then work out, okay, where is this variability? So then therefore, what can we do to help this? But there’s also examples of where it’s not taken into account, where actually there’s been quite big implications of it. So one that comes to my mind is the Ofqual example, which was in 2020, examinations in the UK were cancelled due to COVID for your GCSEs and A levels, so for these qualifications that you do at a higher level at school.
And so the exams were cancelled, so instead they created an algorithm to predict people’s test scores. But then they used those kind of predictions on the model as the individual’s actual score. My understanding of what wasn’t taken into account here is that multi level aspect of the data.
[00:11:35] David: To some extent, yes, but I mean, the thing which I still remember related to that, which is not really about the multi level aspect, but it’s related to it, was that the biases that they introduced just because of what would have been considered trivial decisions, when you round, are you rounding up, are you rounding down? How many A’s should you give? The fact that actually, you’re rounding up means that small schools are getting proportionally more A’s, and small schools tend to be private schools.
So I think, for me, the multi level issue was one, but the more powerful learning that came from that is just the attention to detail that you need if you’re going to be working on a big, meaningful project like that. And, we had another episode around this, about the fact that the RSS turned down that piece of work, and we’re not going to dive into that again because we can point you to that episode and if you want to listen a bit about our thoughts about whether or not you should turn down work if you believe that if you don’t do it somebody less responsible will. It’s a really interesting topic and I could talk about it again but we won’t here.
But I think the point which is I think really coming out is that this complexity and the perceived complexity that does come from having complex data sets, which are almost inherently multi level, this is something where appreciating that and appreciating what you don’t know and even what you can’t know, I wouldn’t have wanted to take on that job because I don’t know that I could have done it well. Because I don’t know that it was possible to do it well.
And this is the sort of thing that ability to recognize, oh, this is hard, this is complex. That’s a skill which is so important, but that’s a skill which could be much more widespread than it currently is. And the perception of people, when I hear people who don’t really know what they’re talking about, feeling, oh, AI will be able to do this or not.
And it’s well intentioned quite often. But it’s complicated because AI is just an analytic tool. It’s an extremely powerful one. But these questions about the levels of the data, if you don’t take that into consideration when you’re actually building those models, when you’re conceiving this, when you’re using it, you’re going to get results which aren’t appropriate, which have biases and so on.
[00:13:55] Lily: Excellent. And so in AI, where does multi level data come in?
[00:14:00] David: That’s a really good question, and it’s one which I actually, I have to confess my own ignorance in a sense. Because, in some sense, the power of certain AI models in the machine learning approaches is that they can identify the patterns which correspond to, if you want, a hidden level. So there is a question about whether they rebuild the structure of the data for you in certain cases. If you have enough data and you have the right data and you have the right sort of algorithms behind it, you can reconstruct some of these and you can find things which actually are surprising and different.
So where’s that balance between actually providing that structure beforehand as scaffolding? And there are, again, AI models that do that. Or, enabling the models to pull them out. And I don’t have any concrete answers to this. There’s a lot of ignorance from my side on thinking about what should be done.
But I think what I do know is that some of the problems are because people are not aware of the structures in their data and so when the models don’t find some of those structures they’re not asking the right questions. They’re not actually then able to pull out things which are or aren’t found and knowing whether to put scaffolding on or not.
So it’s a lot about the decision making around this which is really hard and I’m not saying that there are easy solutions here, but I am saying that too often when I talk to people who work in data science, they’re not thinking about multiple levels. They’re not thinking about the levels in their data and what the nature of the data is enough.
And so I would argue that’s a data literacy issue. So the data literacy, which needs to include multi levels, I would argue also applies to data scientists. And I had interesting discussions recently about what is data literacy. And it really is, I don’t know anyone who has a satisfactory definition for me, so I’m not going to try and give a definition, but what I do believe is that data literacy is not a single state.
It is something which you can improve your data literacy. Just like in some sense, your literacy, I consider myself fairly literate, but I know that I could learn more and I could understand more about the text, about what I’m reading, about the subtleties within text and my literacy could be improved.
And I think data literacy is the same. It shouldn’t be seen as something about black and white, you are or you aren’t. It is about the fact you should be able to improve your data literacy considerably over time and continuously as you build better instincts, better intuitions around data and your understanding of the structures within data and the natures and the things to look for. It’s a continual spectrum.
And so I would argue that the idea of improving data literacy for data scientists is as important as improving data literacy for the general population. And so within this, I would come back to the fact that at all levels, I think multilevel data should be integrated into data literacy, from the very beginning, where people who haven’t been exposed to data, all the way through to data scientists, people who work with data on a daily basis. I think there are elements of that where the tools and the teachings that we currently have aren’t doing enough to integrate multi level data as part of the thinking and the literacy that we just have.
[00:17:46] Lily: Often I think having terms like multi level data is what puts people off. As I said, I didn’t do it in my bachelor’s or master’s, so it wasn’t until I was actually working with you that I realized, oh, is that it? It’s just about having these different levels in your data.
[00:18:03] David: Absolutely. And it’s not just about the term, but if you think about that when you’re exposed to it, it isn’t a big deal. It doesn’t need to be a big deal, but it does change your thinking and change the perspective you have about how you look at data. If you’re asking the question, what level of data, what level does this belong? How, where should I be thinking about this? And so on. And actually bringing that into your thinking, I think it will make a big difference if we actually got more people thinking about this.
[00:18:32] Lily: Yeah, I think it adds a lot of depth as well to the analysis or to when you’re looking at the data.
[00:18:38] David: And let’s just come back to maybe finish on this point about where’s the variability. I do believe that a key concept within data literacy is about understanding the concept of variability and how analysing data is all about understanding variability or, sorry, not understanding I’ve been told off for that. It is about explaining variability, accounting for, no, explaining is bad as well. I’m really not doing well here.
[00:19:10] Lily: Accounting for.
[00:19:11] David: Accounting for variability. And maybe it’s worth just a minute to say why, or maybe we should do this in another episode where we dig into why I’m so bad with my language on understanding and explaining. I think we’ll leave that for another episode, but don’t use understanding or explaining variability.
I used to, I have been told off for that. And the correct term is accounting for variability because that has less confusion. And the importance of accounting for variability is really about being able to recognize that there’s some variability, which is just natural.
There’s some variability which you have accounted for, and there’s some variability in between which you could account for but you haven’t. And a lot of the analytic process for a good data analysis is about understanding, how much can you account for, and what is it you really can’t account for, and what’s left in between, and is that okay? Is that all right? Is your analysis okay? Or are there features within this which are really going to mean that your analysis is misleading or misinterpreted?
And so thinking of that in that sort of way is critical because the variability you are accounting for can’t be understood well, the natural variability, unless you understand that natural variability could be occurring at multiple levels. Just as we said with the school example, it could be the variability between children, could be the variability between teachers, could be the variability between schools. These are all sources of natural variability, and they present themselves differently in your data, but they’re natural.
[00:21:04] Lily: Excellent. Thank you very much, David.
[00:21:07] David: Okay, thank you. This has been good. I think, as podcasts go, it’s been maybe a little bit more of a content focused podcast than our normal discussion based podcast. So I apologize for that. This is a topic which is close to my heart and I appreciate the opportunity to have discussed this.
[00:21:24] Lily: And it’s, I think it’s always useful to break it down to, as I say, multi level data sounds so much scarier than it is.
[00:21:33] David: You’re absolutely right. It is extremely important, but it is not hard. And I think that’s one of the tricks here. This is something where it’s a very simple concept in its essence, which everybody inherently understands as common sense.
But they don’t bring that common sense necessarily to the table when they’re analysing data because it’s not part of the way they’re taught about data. And it should be.
[00:22:02] Lily: Great. Thank you very much, David.
[00:22:04] David: Thank you.