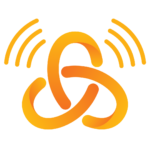
Description
IDEMS co-directors David Stern and Kate Fleming consider the concept of collective intelligence and its implications for society and technology. The discussion covers the relationship to artificial intelligence, misinformation, and how collective intelligence can be utilised to help address global issues like climate change and systemic problems by democratising and elevating diverse forms of expertise. By emphasising the role of marginalised communities in developing inclusive technology, collective intelligence can lead to better societal outcomes and more effective solutions.
[00:00:00] David: Hi and welcome to the IDEMS podcast. I’m David Stern and I’m here with my co-director, Kate Fleming. Kate, it’s great to have you again.
[00:00:17] Kate: Hi David, nice to see you.
[00:00:19] David: Good to see you too. Today we’re discussing collective intelligence, I believe.
[00:00:25] Kate: We are, yes. I brought it up because one of the things in anything in the tech space is that there is a need when you are talking to people outside, particularly when you are fundraising, to think about what are trending topics? What are things that people are thinking about? Where is there validation through research? What is the thing that gives people the lens through which they can understand the value of some sort of innovation. Because otherwise it can feel like it’s not connected to things that are going on, or it’s ahead of its time, whatever it is.
So I think we’ve felt a need to really connect ourselves to a conversation where people are like, okay, yes, I get that, that’s a problem. And so I brought up the topic of collective intelligence because it’s something that the UN has brought up. It’s something that Nesta has written about. There’s a general understanding, particularly around climate issues, but I think around all kinds of issues, I just think it’s that the climate applications are better understood, or the use cases, that collective intelligence can play a very important role.
So, I think part of our conversation is, well, what is collective intelligence? And then also thinking about what is our, our, as in IDEMS, connection to the topic and what role are we playing and do we have to play and does technology have to play? So that’s kind of the big way we came to this topic. Hopefully that’s a helpful introduction.
[00:02:00] David: Yeah, and of course, a lot of people nowadays are talking about artificial intelligence and in many ways collective intelligence is the opposite of artificial intelligence. It really is a group intelligence. It’s a really interesting topic to think about this and think about how it relates to technology and to the way our societies are going and the choices we’re making.
Do you want to give your take on collective intelligence and maybe we can iterate on that or should I start?
[00:02:33] Kate: You could start.
[00:02:34] David: Okay.
[00:02:35] Kate: Unless that’s putting you on the spot.
[00:02:37] David: I don’t mind being put on the spot. I put others on the spot all the time so I’m happy to be put on occasionally.
My understanding of it really is that this is, in many ways it’s related to a lot of ideas that our societies are founded on. The idea that a jury is better than an individual judge is an instance of where the idea of collective intelligence comes, it’s an output of the idea of collective intelligence where together you’re actually better decision makers and you make the right decision more often.
It’s this idea that in a society if we actually have the collective intelligence then we make better decisions. This goes back a long way in different ways, some of these ideas. Democracy is really founded on the ideas of collective intelligence actually being a good way to make important decisions.
[00:03:31] Kate: I guess I would distinguish between the wisdom of crowds, and collective intelligence. Like, collective intelligence is where, in my opinion, perhaps this is open for discussion, but I think collective intelligence is about identifying knowledge sources and wisdom in places and in individuals or groups. Not in crowds necessarily, where I think democracy can often be… Democracy is great. But within democracy, you want experts. You want people who are experts in particular things.
And so I think of collective intelligence as something that is more thoughtfully thinking about how to elevate expertise, but to democratise the elevation of expertise so that you’re not just saying, well, this particular kind of person is an expert and what they say goes.
You’re saying, well, there are all kinds of different expertise that maybe some have just been silenced or not recognised or marginalised, but how do we bring those in and include those? Well, I guess that’s just to distinguish between a more kind of flat inclusivity.
[00:04:44] David: And I guess these are the interesting discussions within that my understanding and my understanding is not perfect on this is that collective intelligence as a concept is actually multifaceted, there’s a lot of different theories which have come out and there’s a lot of different thinking which has happened around this.
And it’s not something which is new, which I think is important. It is something which has a long history. And as you say, there’s distinctions between, I would argue, the wisdom of the crowd is a form of collective intelligence, but it’s not the only form of a collective intelligence. And what you’re saying is that there are other ways to recognise and value expertise within collective intelligence without it becoming about the individual.
And I think this is the key point, you can recognise and value and elevate individuals within a collective in certain ways while remaining within the broad landscape of collective intelligence, is my understanding. And I guess part of the question for us on this is really, why is this a hot topic now and how does this relate to, you know, what’s happening in the world where we are seeing elements of democracy, I would argue, breaking down a little bit where the wisdom of the crowd is not necessarily as wise in certain ways, and experts aren’t being valued because of a wisdom of the crowd, whereas experts have knowledge.
And so there is this sort of tension between, well actually what is knowledge, intelligence, you know, how should we value experts? And as a society, the confusion between information and misinformation, this is a really serious issue of our day, and it’s all tied up in some sense with some of these ideas of collective intelligence, and why I think you made that correct distinction between the wisdom of the crowd, which might be misinformed, and collective intelligence drawing on experts to try and get to good information. And I think there’s some very difficult questions here.
[00:06:57] Kate: Yes. Okay. So there are two things there. One is why now? And I think it’s because we’re seeing these really hard problems where there’s a recognition that these systemic, what we refer to as grand challenges, wicked problems, that these really big global problems, one size fits all solutions, the idea that just a single expert can come in and just deliver something that’s going to work, that’s not working. So that’s a big piece.
And then I guess the other thing that I was thinking as you were talking, and this is something that I’ve been interested in, just thinking about more generally is I feel like we are still riding the wave of enlightenment thinking. And enlightenment thinking was very much about compartmentalising and defining and you create systems and hierarchies of value. So there’s this whole path that we’ve been on where expertise is quite hierarchical and it’s, I would say, it’s designed to give power to certain people.
[00:07:57] David: Can I check, are you really meaning just hierarchical or are you also meaning, you know, it’s this silo thinking about creating different disciplines, discipline thinking?
[00:08:07] Kate: Yes, I would say like the animal kingdom is obviously like a very clear example of that, which maybe it does make sense. I won’t even get into animal kingdom, but we break things into systems, it is definitely siloed and then within those silos, things are defined, they are kind of systematised in ways that allow them to become pieces that can almost be accounted, where everything is an accounting system.
And I think that that has real limits. And I think a lot of people who’ve been on the low end of the accounting system are feeling quite ill done by, where they are recognising they’ve been very ill served by these systems where, for example, if you build a system where a neighborhood is just defined by its poverty, and then that becomes the defining factor of the neighborhood, that misses all of the nuance. And yet a lot of technology, a lot of systems, a lot of programs are built on just the system that’s been built around kind of defining the attributes of economic standing or whatever it is. I’m not explaining that very well.
[00:09:15] David: Let me feed back to you what I’ve heard. I think there is a real element here where what you’re describing to me from a scientific perspective is very much quantitative methods. You’re saying that a lot of what we’ve done is we’re trying to categorise things so that we can quantify them, and this has been a lot of our scientific process for quite a long time.
There was an episode which I believe has come out relatively recently where we’ve discussed actually qualitative and quantitative and the value that quantitative brings and also the value that qualitative brings on top of this. And it’s been a long time that people have recognised that just quantifying isn’t enough. But it’s very interesting that the way that you brought it out isn’t in terms of research methods.
You brought it out in terms of societal structures and societal approaches. And I think that is correct that there has been a lot of effort to try and use scientific process, in particular quantitative methods, to be able to bring order to society and to structure society. And often this has led to decisions which from a human standpoint sometimes just don’t make sense.
But from a purely numeric or sort of quantitative standpoint, well it’s the logical thing to do. And this is where I think there’s a growing recognition, and this is, I think, where the collective intelligence is coming from, that actually with the machine learning coming in, well, this is just exacerbating all of this. This is taking those ideas and enabling us to remove human responsibility even further and go so much faster in that direction.
[00:11:14] Kate: Yes.
[00:11:14] David: That now there is a recognition of, wait a second, we need to bring back this collective intelligence. We need to bring back some of these elements where we actually get human intelligence more involved in some of this decision making.
[00:11:28] Kate: And I think especially the black boxing, that’s a big, you know, term in tech, but that idea that so much is becoming increasingly just kind of built into the algorithms and systems that people can’t see. And even the people who built these systems can’t really say why. So it’s like you have possibly unfair, not always unfair, but just not broad enough to be inclusive systems that are just replicating, they have bias, whatever it is.
But yeah, I mean, you just see that those, foundational values, which should in and of themselves be questioned, have just been taken as givens. And then it builds in values that aren’t necessarily representative values, that aren’t necessarily inclusive values. I think there are lots of ways that systems tell some people that they’re better than other people.
And those systems are maybe just designed to de risk insurance. Or de risk lending people money. But then that actually becomes a very defining feature because it’s taken, so a credit score or something, is taken as a virtue signal, where it might just be down to poverty and you lost your job and you had whatever the system, the things that happened, where there’s a lot of complexity there.
But then that becomes a foundational piece of data. And the assumption is that system is a fair system, that it’s a good system, that it was built by people who had thought through all of the details, and then that just gets extended and extended. And so that’s a very narrow intelligence that’s increasingly becoming expansive. And that, I think, is where we’re finding, one, it just doesn’t help solve a lot of problems because your credit score actually, that’s not helpful information in actually trying to address problems.
And, I don’t know, I had a 2, but I forget what my 2 was. [Laughs]. It’ll come back to me.
[00:13:31] David: But I think the thing which you are getting to here, which I think is really the heart of this, where this relates back to IDEMS in some sense, and what we’re trying to do, and how we can articulate what we’re trying to do, is that if you design systems where the assumption is that you can use data which is out there to outperform human intelligence, which is what a lot of the artificial intelligence systems are now currently designed to do.
We would argue that you’re not using artificial intelligence responsibly, that this is not about removing humans from the loop. And this is a really controversial point within the artificial intelligence communities. What should the role of humans be in the loop when you’re developing artificial intelligence systems?
And it’s interesting that it’s controversial in certain circles, in certain places. But when I talk to mathematical science experts on this, there, there’s relatively little controversy because the experts understand you need humans in the loop. If you don’t have humans in the loop, we’re just doing algorithms. We don’t know what’s going on. We don’t know how to make good decisions. We don’t know what the data is telling us. You know, the algorithms don’t understand the data. They’re not intelligent as we understand human or collective intelligence. We need humans in the loop.
And this is where I think it’s really interesting that we’re getting back to this idea of collective intelligence. What would it look like if our artificial intelligent systems were designed to incorporate collective intelligence in a very different way?
[00:15:24] Kate: And I think our insight is that to even begin to get there, there’s so much for foundational infrastructure that has to be built to enable the inclusion, the participation of people who don’t necessarily look or act like what most technology is built, like the people most technology is built for, who have resources, education, are digitally savvy, all of these different things, have consistent internet even.
And so how do you even begin to bring those people into participation to elevate knowledge that they hold that really is valuable. Maybe they don’t have systemic research knowledge, but they certainly have local knowledge. You know, they will have an understanding of why props failed one year that might not be based on the science of climate change, but will bring in variables that are down to local factors that are helpful for researchers to be factoring in, even if it’s to realise these actually weren’t relevant to what happened.
[00:16:30] David: But I think, that particular getting down to local knowledge is, I think, critical. I want to maybe just step back in what you were saying there, that we don’t have the answers to this. And I want to be really clear on that yet. This is a hard, hard problem. And this is a societal level problem, which we are facing right now.
I believe that as a society, we should be looking to build the artificial intelligence of the future based on integrating collective intelligence in important ways. I don’t know how to do that and I don’t believe anyone else really knows how to do that. I know instances where we could do this, and we could dig into some of those examples.
[00:17:15] Kate: Yeah, you should dig into it right now.
[00:17:17] David: Okay. In the past, one of the examples I’ve used with Responsible AI where I’m concerned is birdsong. And this is an instance where, you know, I don’t see how we can build systems where you get the right feedback loops so that you automatically get improved identification if there’s an evolution in the bird song, and so on, that this naturally happens.
And talking to experts in this, they agree, it’s all about having well trained humans in the loop. Now, it just so happens that for birdsong, there’s a whole community who are passionate about birds and who get trained on identifying birds by their song and in different ways. And so there is this expert community that therefore needs to be central to anything that gets built in the future and how you do it.
However you’re doing it, it’s about actually this pairing of this community of human experts able to identify and, you know, passionate about birds and to get them to work with the artificial intelligence systems, which can then enhance the fact that their identification skills can now be scaled to anyone who wants to identify a bird at any given point in time and get it right most of the time.
And if you want to do conservation, if you think about conservation just on the artificial intelligence, you run the risk of over time, it getting wrong, it going in a wrong direction. But if you pair this with experts who are part of that conservation effort, and who are actually validating, it’s about that community.
And this is your collective intelligence. It’s not about an expert, but a community of experts who hopefully argue amongst themselves and say, no, this is new, it’s a new song. It’s a different song to the previous song. This is a wonderful example in my mind of where artificial intelligence is so good at helping us to use that expertise of that community for a much wider community. But without that expert community it doesn’t go anywhere.
[00:19:45] Kate: Yes and even within that I think there’s also the recognition that there is a technology stack there that’s also interacting with the humans. I think we both went to the same talk. I’m going to forget his name of your professor.
[00:19:59] David: Tom Denton.
[00:20:00] Kate: Yeah. Actually, he’s at Deep Mind.
[00:20:03] David: Yeah.
[00:20:03] Kate: I think, is that?
[00:20:04] David: That’s right. Yep.
[00:20:05] Kate: But one of the core things was also just the acoustic technology. So that idea that you can pick up sounds so much more… One of the recording technology, so just to be able to record for long stretches of time, two the acoustic precision to start to see, when he was showing, we would listen to a bird song and it sounded to my human ears, like maybe three notes and it was kind of not, whatever. And then you see the visualisation and you realise, oh my gosh, there are all kinds of variations in there that we’ve, as humans could never have heard that show that there’s a lot of distinction. There’s more happening here. These two calls that maybe sounded quite similar had variation that birds could quite easily obviously pick up.
But you see the constant interplay, I think of layers of technology innovation that’s also required. Like the idea that we just step into some AI world where it’s like the data just exists and then it just gets processed and whatever. There’s so much that has to be happening all the time between humans and actual technological innovation to even begin to step things forward. So even that is a form of collective intelligence where the ongoing innovation intelligence that has to be happening to be solving for different problems, that’s a big piece of the collective intelligence.
[00:21:30] David: Absolutely, and this is where, if you think about our priorities, and I think you drew allusion to this before, our priorities are often those who are not currently being heard. We really work in the lowest resource environments with those who are not necessarily digitally literate. They certainly don’t have a big digital footprint, which means they’re not part of the big databases. Their voice is not currently loud within the AI models which exist because there is no data on them.
Why is it so important to work and to sort of focus our work on those communities at this point in time? It’s not because we have the tools at this point in time to elevate their voices and to get them part of these big AI systems. No.
But what is happening right now is that such people can benefit from technological innovation, which may or may not relate to AI. There are instances where I can see AI being useful, but they’re small. It is just the technological innovation. I love the fact that you’ve got this sort of multiple technologies needed and being able to work in those lowest resource environments.
My claim is that actually the learning from there is going to help us to build out this collective intelligence in a way which we can serve those communities better because they cannot be exploited in some sense. They need to be served because there is nothing to extract. And understanding how to serve community is the big challenge that I would argue we have with technology.
[00:23:14] Kate: Well, there is something to be extracted, but I think we want to think of it as like gifted or something that’s reciprocal as opposed to what so much data gathering has been perceived as. It’s just like, how do we get at this and take it out and do whatever we want with it versus, you know, how do we start to collect this data, make people feel that they have ownership and input and say in what looks like data and insights. And begin to join in something that is a more reciprocal exchange of value and standing. Because I think there’s such a power dynamic right now, the way technology is built, the way our systems are built.
So if you are in an underserved or low resource community, your experience is mostly people just come in, they take. They maybe solve stuff, help you with something for a little while, but then they kind of disappear and whatever. That’s a big generalisation.
[00:24:13] David: That’s a big generalisation. And I think, the thing is, to avoid those big generalisations, I think what is true, is that most technology, technology companies, their bottom line is profit. And because the bottom line is profit for technology companies, they aim to put themselves in the middle of transactions and be extractive. Because that’s how they extract their profit. So this is not a criticism of big tech because actually being in the middle of the transaction doesn’t mean that you’re not smoothing the transaction.
I’m afraid credit card companies all over the world have helped ease transactions all over the place and reduce friction, but they’re in the middle. And so they are taking their little bit of profit in the middle. And this is what technology has tried to do in general. But when you’re in a context where there is nothing to extract, they’ve been neglected.
And understanding how you can serve those communities so that they can use the benefits that we’re getting from technology, even though there isn’t necessarily a direct extraction to be had. I believe that that gives us the opportunity to imagine other ways of tech being developed so that it is not developed to sit in the middle and extract, it is developed to uplift and to sort of support.
And there’s been wonderful examples over time, open source software in general, where this has then out competed what were traditional approaches, which were protecting, and which were trying to make sure you protected your place. And my claim is, and I don’t know that this is true, that actually if we do learn from serving these marginalised communities, that we’ll actually find ways that our technology can better serve the wider community.
And this comes back to this collective intelligence, that instead of taking a dominant narrative, we’re taking the marginal narratives and we’re forming that in to a more complex patchwork, which is coming through this sort of collective intelligence, whatever that may look like. I think that’s where there are possibilities.
But we don’t yet know how to even build the systems to make this possible. And that’s what’s so exciting. We’re learning bits of this from some of the work we’re doing in different places. And it’s really exciting to me that we keep coming back to the same fundamental problems. That when you’re working for groups that are not your mainstream commercial group, every group is different.
And so you have to be building in a very different way than if you were just building for a commercial mainstream. And that, to me, is part of what actually, I think, could really lead to us benefiting from and building out these ideas of how technology interacts with the collective intelligence, where different voices get elevated in different ways based on how they can contribute locally and how that local contribution can lead to sort of more global knowledge in different ways.
We don’t have the solutions to this, but I do believe there are forms of collective intelligence where you can be listening to the margins and actually drawing that out into something which through technology, supported by good technology where you could be, I think, not just serving the margins, but serving the whole much better.
[00:27:57] Kate: I think what I hear and what you’re saying is so much of this is about unlocking untapped knowledge. And there’s a recognition that it’s almost like an entire natural resource that’s just been kind of unidentified, unchanneled, untapped for its potential to kick things to a next level.
And then there’s the work of how do you unlock that? And a lot of that is, as we work on that, we realise it has to do with developing technology that doesn’t depend on high skills or professionalisation or specialisation even. This is where we talk about collaboration all the time, you know, multi directional, trans disciplinary. I don’t have to be an expert in one thing to be able to work across things to benefit from information, all those different things.
So I think that instead of unlocking profits, it’s unlocking knowledge where it’s like, how do we start to bring that in? And that obviously, when you think about collective intelligence, it is about creating ways to bring knowledge streams together in unexpected, never before, maybe in small ways before, but at scale, how do you bring things together to generate innovation, new forms of impact, new solutions, all of those kinds of things.
[00:29:21] David: It’s really interesting how you frame that. And I think there’s something very deep in what you’ve said, which I’ve not yet taken in, because one of the key things in the choice of the word knowledge in this is that information and misinformation are going hand in hand right now. Actual knowledge, I would argue, is validated information. It’s information which has been now, turned through whatever process it is into something which has been validated.
And that process of validating local information into local knowledge, scientific information into scientific knowledge. Part of this is about weeding out the misinformation because misinformation doesn’t translate into knowledge.
And so that big challenge of being able to sort of work at scale, where you’ve got that valuing of non experts, one of the things about experts is in a particular discipline, your expertise sometimes protects you from misinformation. But what’s happened actually in our societies is that it’s not quite as simple as that. Misinformation has now spread so far and so wide because there isn’t protections on information.
So understanding how we can actually get good knowledge to spread and to be shared is one of the big challenges of our time. And, you know, we don’t have the answers to that, I had a wonderful colleague who 10 years ago now was really worried about misinformation then. And it’s just got worse and worse since. And he’s been working on it. And it’s hard. Misinformation is such a hard topic. And to think about actually reducing the role of experts is very scary for someone who is worried about misinformation because experts are part of the protection you have against misinformation.
But I think you’re absolutely right that if we use that protection then we’re losing voices. And a lot of what we’re trying to do is to try and put in place structures where there can still be systems in place that convert information to knowledge as one example, or that protect against misinformation, or that build aligned with principles or good practice but that support local adaptation, even from people who are not experts and don’t hold that high level expertise. It’s a hard, hard problem.
[00:32:13] Kate: I think so much of what you’re getting at is trust and distrust too. I think a lot of what’s happened is that experts have become so far removed from communities that there’s a lot of distrust. And because sometimes expert decisions haven’t helped people, or just have been part of some suite of policy, and in that suite have been things that have harmed people, even if one piece of it didn’t harm people, or just didn’t take into consideration their needs or their problems.
And so there starts to be this broad distrust, and then in that distrust, there’s so much room for misinformation. And I think this is on the information level, it’s why people who really care about journalism will say right now, the big need is for really good local journalism that holds local officials accountable, local experts accountable, that really does that work, because it’s something that in your smaller network of trust, it makes sense.
It’s like you’re willing to accept a truth from someone who’s in your community of trust, whereas maybe you’re just more skeptical of something that’s remote and far away, or mostly you’ve just seen those remote far away people are just mostly trying to get their own and maybe you don’t feel that they’re that concerned with your well being.
And so yeah, I guess I would identify within what you’re saying, a lot of it is trust. There’s a lot of trust happening in there somehow.
[00:33:40] David: Well, I agree and it’s about trying to build systems where that trust can be built up. And I do like the example and the way you brought out this illustration that experts being distrusted because actually their expert opinion has led to negative impact in so many instances. And a lot of that comes back to where you started with the expert knew something about the numbers.
But there was subtlety, there were details, and this might have been something which on the face of it was a plausible scenario for a large part of the population, but maybe a minority was therefore left behind. And this is what’s happened in so many different instances where minorities haven’t been able to trust the whole.
And I’m going to just mention some of this related to medicine because the gender biases, for example, for heart attacks, the fact that female symptoms of heart attacks were just not reported and therefore were totally being misdiagnosed because they were different than the male symptoms and all the tests were happening on male symptoms and all the descriptions. The fact that it took so long for this to even be identified, that there was that gender bias, it should have been a real wake up call for us in terms of thinking about, well, how should we be developing our knowledge as a society?
And too many of these examples have happened over time, which have eroded trust in the experts who are specialists. And they may be a specialist, but does that specialist mean they’re right for you? Quite often what you need is somebody who’s less of a specialist. But the value within society has often been put on these specialists. And that sort of expectation therefore, that comes with that, this idea of specialism versus generalists, who have a broad knowledge and who are trying to understand what’s falling through the gaps, our society doesn’t put enough value on that.
And this is the sort of problem that fundamentally, if we come back to a lot of the work that we do, we don’t value local knowledge over expert knowledge. We value both. And it’s about understanding how to get that interplay where the experts can be appreciated for their expertise and their expertise can be put in context and cannot be overreaching because it’s the overreaching of that expertise which then leads to these misunderstandings.
[00:36:37] Kate: Yeah, and I would say that it’s becoming very clear that our best collaborative partners are people who bring humility, that we work with experts who really, they are experts, they are brilliant, but they’re also so willing to acknowledge there’s so much they don’t know. There’s so many variables at play that they don’t have research on, that they can’t say, you know, how that variable might affect the application of their research. So, well, let’s try it and see what happens, let’s quantitative and qualitatively try to study that. But I think that willingness to acknowledge there’s so much you don’t know, and to let people who might hold information that would help you know, step up and be equal partners in that moment where you’re trying to solve that problem.
I think most people have been taught that that is not what experts do, that if you’re an expert, you’re right, you know, you just aggressively double down. And it’s like, I can’t create space for uncertainty or for other forms of knowledge. And I think so much of what collective intelligence is trying to get at is kind of breaking down that idea of what expertise is, and the idea of an expert, and really create something that’s more expansive, that is recognising that however brilliant you are in one channel, one is incredibly ignorant in other areas, and to let someone else step up and fill that gap.
[00:38:05] David: Well, this is a great place I think for us to finish. I’m conscious it’s been a long episode.
[00:38:10] Kate: Oh yeah.
[00:38:12] David: But it’s a great place for us to actually finish because I think you’re absolutely right that one of the things that I find is that our systems, our academic systems, often they are competitive. And so people need to sort of impose themselves. But the true experts that you know, as you say, they’re often the ones who have demonstrated their expertise and now are in a position where they can recognise they’re ignorant. And the more expert I become, the more I recognise how little I know compared to what’s out there.
And this is the key that I think, recognising that there are limits to your understanding, that you have knowledge, you have understanding. You have maybe understanding which is more than many of your peers. But recognising more than anything, the limits of that understanding, and where that understanding fails, and what else there is to learn, that’s what real expertise is to me.
As you say, this leads to the idea that actually working in transdisciplinary teams where you build on expertise from others and knowledge from others, is good. And that is another form of collective intelligence and a wonderful place to finish. So thank you.
[00:39:34] Kate: Yes, thank you so much, David. This is a good conversation. I feel like we could continue talking for a long time about this, but yes, good place to end.
[00:39:43] David: Thank you.
[00:39:44] Kate: Thanks.