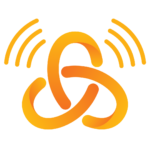
Description
David Stern and George Simmons discuss the concept of collaborative modelling and its transdisciplinary nature. Considering examples from various fields, including agriculture, ecology, and disease modelling, they highlight the need for better communication and collaboration among experts from different disciplines.
[00:00:00] David: Hi and welcome to the IDEMS podcast. My name is David Stern, I’m a founding director of IDEMS and today I am here with one of my colleagues, George Simmons, who’s an Impact Activation Fellow who works on a collaboration we have with the Global Collaboration for Resilient Food Systems and CASAS Global on modelling. And that’s what we’re going to discuss, I believe.
[00:00:30] George: Sounds great. Hi David, how are you?
[00:00:32] David: I’m doing well, how are you?
[00:00:33] George: Yeah, very good, thank you.
[00:00:36] David: So, collaborative modelling.
[00:00:38] George: Yeah, so I’m very interested in getting your thoughts on collaborative modelling and what it means in, well, both the general context and what it means specifically for us. I suppose the best place to start is collaborative working is nothing new. We work together on GitHub. We work together on Google documents and sheets. So what makes collaborative modelling such a different thing in your mind?
[00:01:07] David: Well I don’t know that it is so different and it’s one of those things that when you’re hearing collaborative modelling you’re probably hearing something different to me because you’ve been talking to the Topos guys more than I have.
You know we love the Topos guys and we love how they’re thinking about this. And they’ve got this concept of collaborative modelling, which is defined more formally than I would think of collaborative modelling. So, maybe a place to start there is for you to just say a little bit about what, before I go into what I understand as collaborative modelling, what do you understand as collaborative modelling?
[00:01:42] George: Yeh good question. So for me collaborative modelling is… Well, I mean, you say it could have a technical definition. To me, it is the process of being able to capture a model which naturally uses the expertises of the different people, the different modelling approaches of different people.
It’s a way to combine different modelling techniques, whether that’s differential equation based modelling, whether that’s correlative or statistical based modelling, whether that’s agent based modelling, and, being able to kind of construct a model, which can use all of those components together in a natural way.
And it also extends down to the data you put in as well. Different people have different ways of capturing and storing data and different expertise areas of the data they’ve come from. For me, it’s being able to kind of capture all of that together.
[00:02:33] David: Exactly, and this is exactly where I hear in what you’re describing, what I understand of the Topos approach to collaborative modelling, which I really love and I buy into. But inherently in what I hear is this transdisciplinary nature of the modelling that you’re describing. It’s the fact that you’re modelling multiple processes. It’s the fact that there’s actually real complexity to the modelling you’re doing.
And fundamentally, most modelling is simple, it is a single process that people model. And these ideas are the needs for these bigger models, which model complex systems, I would argue this is newer, and this is what Topos is getting involved in.
So, working collaboratively on a simple model within a domain for a single thing, people have been doing that for years. And that’s different to what you’re describing. Now, would I call that collaborative modelling when in the past I would have? This sort of working together, and there were lots of big models on this. I mean, the big climate models are incredible. We work in agriculture and the crop models, APSIM, DSSAT, these big crop models, they’re incredible. And they’ve been worked on in really collaborative ways, but they would not satisfy your definition because they don’t cut across different modelling techniques or disciplines in quite the same way.
Actually they do, sort of, but they’re not built in this approach that you’re describing. And the approach you’re describing is one I really like. And so the idea that we can give a definition to collaborative modelling, or to a collaborative modelling approach that is more transdisciplinary, that looks at this, taking this from multiple perspectives.
Well of course the reason Topos, the way they think of it comes from this is because they’re coming from a category theory perspective. Which is something which as mathematicians we like, not all mathematicians like category theory, but we both do. So it’s something which comes very naturally to us. But it’s not very natural to most modellers.
Most modellers wouldn’t think like that. They would be thinking about building a model and building out a model. The collaborative modelling, as you’re describing it, and as I would argue, Topos are working on this approach, is one which is inherently bringing in multiple perspectives or multiple views on the problem and on how you would do the modelling, how you would source the data, what types of systems you’d use, or how things could therefore fit together, where people look at their bit from a different perspective, but they fit into a coherent whole.
And that’s what I understand when you say collaborative modelling. But it’s not what comes to mind for me when I think of collaborative modelling. Does that make sense?
[00:05:38] George: Yeah, to kind of summarise what you said, it’s really the way where you can capture the benefits of a transdisciplinary approach in building up your system. I think that’s a very important view.
[00:05:51] David: And therefore, in what you’re describing, I understand how this is different to, as you were saying, working on a Word document together, or Google Docs, or a Sheet, or whatever it might be. Because that corresponds much more to the sort of modelling within a discipline, within a single view.
What I would argue is that what you’re describing, this exists a little bit in other contexts. Let me explain what it’s not first, but it is almost. So let’s say some people are working on a Word document, and other people are working on a spreadsheet, and other people are working on something else. This is all coherently coming together so everybody is actually seeing and working on the whole.
Now, when you think of it that way, this feels very, well, how can that be? Because the whole, is it a document? Are you embedding the spreadsheets into the document? Is it a spreadsheet? Are you just having the things that people worked on in the document in sales in the spreadsheet maybe or something like that? If you’re looking at it from one of these perspectives, this doesn’t really make sense.
And that’s why the parallel doesn’t really exist in that same way. But that’s the parallel I can give for what we’re doing in terms of that modelling approach. Imagine one person is working on documents and another person is working on spreadsheets. And yet they are both seeing and working on a coherent whole, which includes everything the other one is working on.
Now you can’t really do that in other areas, but in the modelling you can. The tools to do this, I would argue, are still in their infancy. And Topos is thinking about this, and they’re thinking about how we build models in this way, to have this in a coherent whole. But that approach of being able to have those multiple really totally different perspectives, where people are working on it in totally different ways, contributing to a coherent whole, oh, that’s interesting and exciting.
And I think it’s because of the mathematical nature of the models that you therefore get these different views, which can be represented in different ways.
[00:08:01] George: And that’s very interesting. I know as mathematicians, we do focus on the mathematical structures that can kind of get us there. But this is also about understanding how to communicate between those disciplines, the different people who come in to build a model. And this is exemplified by the work we’re doing with CASAS, that we are mathematicians and mathematical modellers, and they are ecological, they’re biologists, they’re entomologists, they’re ecologists.
[00:08:30] David: They’re also modellers. It’s really important to draw that out, they’re creating more complex models than pretty much any mathematical model I’ve ever seen.
[00:08:38] George: And finding the way to, you know, the first step to be able to model collaboratively is to find the ways to communicate the ideas between yourselves, and I think that’s something that’s been a great challenge in building up the collaboration that we’re doing with CASAS. But also something that just opened so many doors, because it’s allowed us to take their ideas and think about what they mean and that slight more bit of generality, which allows us to actually implement them.
I’m wondering if you can think of any more examples of situations like that you’ve come across where you’re trying to…
[00:09:15] David: I mean, the case really, which has sort of been highlighted by Topos, and I know I keep coming back to them, but I think their work on this, our work has been inspired by their work. And my understanding of the case that they’re really looking at is really disease modelling. That’s sort of one of their main motivating cases. They have others, of course, but really, this became apparent as a need really through COVID when actually, mathematical modellers were drawn in to work on disease models in a way which was rather different to how they would have worked on them in the past because of the urgency.
I will tell a story that I heard during COVID when, let me give a few details as I can while actually still being a sensible story, I’m afraid. There were a set of expert mathematical modellers who were meeting to discuss how they could help with the modelling required for COVID, and they were joined by medical doctors who were at the front line in hospitals, trying to face the onslaught that was COVID at that point in time.
And my understanding was that the doctors offered the data and the immense amounts of data that were there in the hospitals saying look we just need help because there must be things to be found out and understood from the data we’ve got and the data we’ve got we can’t make sense of it. Surely, having the models to look at this and be able to gain insights from this, what can you do to help us understand and improve what we’re doing, and so on. And the mathematical modellers in the room said, no, that data isn’t the sort of data we need for our models.
And this is so typical of a mathematician led approach to modelling. I’m not saying that the models that the mathematicians work on aren’t useful or aren’t valuable as a mathematical model, you know, but what I am saying is that that approach which comes I believe from a real problem that we have more generally in scientific research of disciplinary priority over transdisciplinary priority.
Not enough people are used to working across disciplines. That opportunity was lost. And part of the reason that opportunity was lost is because, actually, it would have been incredibly hard. The mathematicians were right. The models that they were working on would not have helped in that situation in their current form because they weren’t built to help that.
And actually that type of model, fitting it in with the models they were doing, this would have been really complicated and so on. And so it’s not something which would have been really possible. So this isn’t a criticism at all of the mathematicians, but it is a criticism of the state of our scientific research.
How is it possible with the advances we’ve had, that we are not better at being able to sort of work collaboratively from different needs on perspectives on something as important as that? And that, my understanding, is this is what the collaborative modelling approach could do in that context. Done right, it would say, great, you have that information, and we could then work with you. It’s not our core speciality, but we could work with you to have people who help you to put that into these sorts of models around what you’re doing. And then we could interface that with the models we’re building of the disease, the pathogen, and how it’s being transmitted and what’s happening.
And we can interface these two to see how, as the disease evolves, how it might affect what you’re needing to put in place and how you need to work. You know, that’s what we need to be doing. We need to actually be able to collaborate on really difficult questions and difficult problems.
We shouldn’t take credit for this insight. Other people are thinking about this and I believe that COVID highlighted to some of us, I don’t think I had that insight before COVID and therefore the need for this collaborative modelling approach. So some of us have really learnt through some of the experiences we’ve observed in COVID how we need different scientific methods, almost, to build models in a way which can serve either in emergency or just these more complicated problems.
[00:14:32] George: Yeah, that’s a really interesting thing you brought up, and me speaking as a mathematician is really just you know, branched out in the past year. My exposure to data doing a PhD in pure mathematics was nil.
[00:14:49] David: Yeah.
[00:14:50] George: And ultimately being thrown into this modelling work, I would have no idea how to take, for example, a field study where you’re counting the leaves of a plant or you’re estimating the number of insects on a particular crop and actually translate that data into a useful model.
That’s the big skill that the collaborators at CASAS have, they understand what it means to conduct those studies, conduct those experiments, and use that to estimate good parameters, which are useful in models. And I think that kind of ties into exactly what you’re saying there, is there’s all this data available, but sometimes, well, from the perspective of mathematicians, we may not know, what to do with that at all.
And you need that expertise of someone who understands the ecosystem or the disease or the other complex system you’re trying to work in to kind of lead you on how to take that data and make it useful in terms of making a model.
[00:15:56] David: Absolutely. And I think one of the key things that you’re highlighting there is this fact that as mathematicians, we’ve got the easy job, you know, actually building the models for the underlying systems, this is great and it’s really important. And if you don’t have good mathematicians doing this then your models have features in them which are determined by the mathematics which is used instead of actually representing the data which is coming out and so on.
Don’t get me wrong we play an important role here. But our role is relatively small and I would argue less important than the role played by those who have the domain expertise to be able to build good models. And the key is that in some sense, and we get this every time we talk to sort of good modellers in pretty much any discipline, is that the actual accuracy of the model, the fact that the mathematics behind it leads these sorts of features probably doesn’t matter that much in many cases.
It matters to me. It hurts me when I use crop models and because of the way I subdivide my soil, I’m not actually changing the soil, but I’m changing the mathematical representation of the soil. And that changes the impact, how the plant grows. That’s obviously bad. I’m obviously doing it wrong. So it hurts me as a mathematician that, you know, the way the model is built affects the model itself. And that just means that the maths isn’t right behind it.
But as a modeller, why doesn’t it hurt the people who are experts in this? Well, they say it doesn’t really matter to me, because I know how to use the parameterisation which corresponds to the realities on the ground. The example I’ve just given is about water penetration and the way you subdivide the soil relates to how fast the water goes down in the soil and penetrates the soil. And so they actually have pretty good senses of that from understanding the soil profile.
They have a pretty good sense of what the water penetration rates would be. So you’ve got a discrete model, which is a daily model, and you’ve then got these discrete soil layers. So you just need to understand that things map onto your two discretisations, and that they’re mapping onto each other quite well.
As an expert modeller, the experts are building these models, they do that incredibly well. But it does make it very hard because you have to have that underlying knowledge and understanding of the relationship between water penetration and the time and how the plant is growing.
And that’s something where that expertise as somebody using these models, we want to lower that because when we’re talking about collaborative models, well, if I’ve got a soil science expert and I’ve got a sort of an entomologist and a plant science expert and so on, I don’t want them to have to worry about each other’s domains. I want them to be able to put in and use their expertise and for the maths behind the scenes to be able to present them the views on what the other has done so that they don’t need to worry about it.
And the compatibilities, you know, we’re not going to get these mathematical artefacts. We’re going to get actual easy compatibilities. This is hard. Don’t get me wrong, what we’re trying to do with collaborative modelling in many different cases is hard. But if successful, it could transform who could become involved in using and making the most of these models, because you no longer need your subject expert, who is also an expert modeller, in quite the same way. You actually would get people, it would be more accessible to people who only have the expert knowledge and are less of a modeller.
Now, my hope is that actually those who are expert modellers, what they would then do is they would migrate to being a better interface with the other, you know, components. They’d be sure to become more transdisciplinary because they’d understand the underlying structures in the model much more.
So, the big idea of collaborative modelling, not just as people collaborating on models, that’s too simplistic, but as you’re defining it, and I would argue Topos is defining it, of having these complex system models, which include multiple perspectives and views, which require different subject expertise to come together to contribute to a coherent whole, that form of collaborative modelling, oh, building the tools to enable that, that’s exciting. That’s what you’re working on, I so enjoy this part of my work when I get to contribute to this.
[00:20:58] George: Yeah, it’s an incredibly exciting thing. And I think what you just raised there, this is not just about building the models, it’s using them. And that’s an incredibly important thing, and one of the most important things there is when you’re trying to take away the necessity of that subject expert to actually run the models, you require that level of trust in what you’ve built. And for me, at least one of those ways is being able to attach everywhere you’ve done the right citations, the right track records, the right, history of where your data or assumptions have come from.
I maybe just want to pick up on that data because a collaborative approach to handling and manipulating and tracking data is something that we’re also trying to work with, again with Topos, is that right?
[00:21:55] David: Well, with Topos but I think more generally that this sort of data piece is one which, this is what we’ve been doing more. They’re now interested in what we’re doing there because of the parallels. You know, the collaborative modelling and the collaborative data piece, actually these aren’t so different. And of course, this has real implications in all sorts of places.
Most people are only interested in this recently because of the applications towards AI, and how this collaborative data approaches could transform AI so that we could actually do, well, we could build collaborative AI rather than it only being able to be built by people who have access to everybody else’s data and maybe own other people’s data.
If we have collaborative approaches to this, we could actually have built these sorts of powerful AI systems in more collaborative ways. And that could actually have communities owning their own data and collaborating towards something bigger. Of course, that’s a whole nother discussion. I don’t want to get lost into collaborative data for AI, and that’s in its infancy again.
But the more general process of actually being able to have data which is coming from multiple sources, serving multiple people, being used in different ways, in a way which is really useful. Well, the underlying structure for that is something which is well understood and it’s a database. And that’s been around for ages. And so in some sense, the tools for some form of collaborative data, well, that’s not new.
But the way of that working and the tools to be able to do so in really collaborative approaches, most databases are owned by an individual or an individual organisation, that ownership of the database is important. What happens when you have databases where no one owns the database. You have components of it owned by different people. Then you need whole sorts of protocols to be able to say, well, how are you exchanging data across the database, across the whole, what is that whole, where the ownership of the components are different?
And it might be the ownership of pieces of the data is different. Or it might be that the ownership of types of data are different. Now you’re getting into this sort of idea of really collaborative databases in different ways, it’s all about ownership.
And these are things where this is really fun stuff and hard, but it’s really important. And I think it is something where there was a growing recognition of this importance in a small niche circle, because most people working with data at this point, are happy with the single ownership.
But the parallel is there, that you’ve got with this modelling. It’s the sort of having multiple views with different ownerships of pieces in different ways. That’s the connection. And this is the collaboration we’re looking for with Topos, because that is hard.
IDEMS as an organisation is relatively young. And we’ve already always had these ideas at the back of it and these sorts of things that need to be done, but we’ve always been too small to really take on the more research, mathematical research components. But what we’re doing is building out the use cases so that we can justify that research which otherwise is not being prioritised.
Anyway, this is exciting stuff, that collaborative data, collaborative modelling, this is something where we could keep talking about this forever. We’re coming to the end of our time. Do you have any last thoughts or questions you’d like to sort of finish on?
[00:25:38] George: I guess the only one that’s come up is, you talked about the shared ownership of data, how does that kind of extend to that parallel with the models? Because there is always some sense that the person who’s created that part of the model, that’s still theirs in some sense, they still could have the right to license that. So how does that kind of all fit in together?
[00:26:03] David: That’s a really good question. And I don’t have the answers to that right now. But what I do believe is that, with open licenses, this is easy, you can separate out the ownership from the, if you want, the usage and the adaptability and all the rest of it. So, with open licenses, it’s easy, but you don’t need to just have it for open licenses.
And there’s ongoing discussions about alternatives or sort of ethical licenses, which may not be fully open, but protect people in different ways for their intellectual effort. There’s a lot of interesting questions around that. And I believe as we go forward, one of the ways we’re going to be able to move forward those ownership discussions is by having the tools which enable us to work across different ownership and different licensing models in ways which are powerful and compatible.
Let’s say, for example, that, well, we’re going to get lost if we spend too long, but I was just going to give an example where you have a part of the model where a simple variant of this model is open. Somebody puts a lot of work in and they now release a variant of the model which is better in specific contexts, but could just be plugged in but is not open.
And so therefore people who want to use the model in the simplistic way, they can just use the simple variant. But people who then can afford to or need that particular part to be done in this sort of better way, whatever that means, well, there could be a license fee or whatever it is.
I’m not saying this is how it will work, but I’m saying this is absolutely conceivable within a collaborative modelling framework, that there isn’t a problem conceiving the ability to have layered modelling, which would include paid licenses, where if and when you need that level of model, whatever that means. So that’s absolutely conceivable.
[00:28:09] George: Yeah, that’s a great observation, I think, to finish on, of how this could all feed into supporting business plans around perhaps. Yeah, I think we’ve reached our time. So, thank you very much, David, for your insights, comments, and the conversation.
[00:28:23] David: Well, thank you. It’s been great fun.