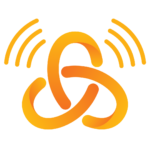
Description
In this episode, we explore the future of AI in education through a discussion with Lily and David, focusing on the potential and limitations of AI technologies. They break down the future into four key categories: tasks that AI will never achieve perfectly, tasks that are a matter of “when” not “if”, transformative innovations on the horizon, and areas with substantial unknowns. The conversation includes examples like the challenges of AI detecting AI-generated content, the potential of AI-based personal tutors, and the complexities of maintaining historical accuracy while promoting diversity. This is the fifth episode in the Responsible AI for Lecturers (RAILect) series on the IDEMS podcast.
[00:00:00] Lily: Hello and welcome to the IDEMS podcast. I’m Lily Clements, a data scientist, and I’m here with David Stern, a founding director of IDEMS. Hi, David.
[00:00:07] David: Hi, Lily. I’m looking forward to our last RAILect episode.
[00:00:12] Lily: Yes, yep, our fifth one in this responsible AI for lecturers, or RAILect, series, and this one is on looking to the future.
[00:00:21] David: Yes, and really, in this “Looking to the Future”, we’ve tried to frame things in terms of broad categories of what the future could bring. Is it worth me going through the four broad categories that we’ll then dig into in this episode?
[00:00:37] Lily: Perfect, let’s do that.
[00:00:38] David: So the first category is one where whatever advances there are in the future, and there will be substantial advances, we don’t think it’s going to make a difference. This is something where statements we think we make today will be equally valid into the future. There’s particular things that we can draw out for lecturers that we think could be useful.
The second category is a recognition that there are elements of AI where the tools that currently exist are conceptually sufficient to achieve things which at the moment we can’t currently achieve. And so it’s a matter of when these sorts of things will be possible, rather than if, in my opinion. And we’ll give one or two examples of this where there is no reason why in the future this shouldn’t be achieved, but there’s good reason why it’s not achieved now, because it’s too hard for now, but the tools that we have mean that we just need to chip away at the problem and we’ll eventually be able to do it. So a matter of when, not if.
[00:01:56] Lily: Nice. And then the third way?
[00:01:59] David: The third is that there are areas where innovation is needed, but we’re expecting it to happen. And it’s going to be disruptive, and we don’t know what it’ll mean, but there are indicators already of areas where this could be happening with respect to AI, and that will be transformative again in terms of what’s possible. And so we’ll dig into one or two examples of this. In particular things where, if these big advances happen, or if these, it’s not these big advances I’d argue, because in some sense we’re on the verge of them already, if we’re able to solidify some of the advances which are in the process now, then this could change again what the systems that are being built can do.
[00:02:45] Lily: Interesting. So these kind of breakthroughs that are coming.
[00:02:49] David: Yeah, breakthroughs that I would expect to be coming and how I would expect that to be able to change what we, what these systems can do.
[00:02:59] Lily: Nice. And then how about the fourth way then? So we’ve got things that kind of are never possible things, which are a question of if and when things that are on the verge…
[00:03:08] David: We’ve got things which are never possible, things that will be possible, it’s just a question of when, things that, if certain things happen, that will change what is possible, and that’s an if I would put, and then the final one is, there’s areas where, I would argue, it’s not known. It’s not known yet what will be possible or what might be possible, so I would argue there are still substantial unknowns about things which could potentially fit into different categories. But as far as I’m aware, there isn’t the depth of knowledge yet to be able to know for these instances where will this lie? And we’ve got at least one or two interesting examples of that. Should we dig in and start at the beginning?
[00:04:00] Lily: Let’s start at the beginning. So the ones that you feel are never possible, which is quite a bold statement.
[00:04:05] David: The one that I want to draw out for lecturers here is the idea of using AI tools to identify when a student has submitted something, which is AI generated. And of course these tools are already out there, they’re being used. And they have problems that we’ve identified and we’ve talked about, the Reading study, where two out of 33 were identified. I’m not saying that there aren’t tools that could do much better than that, but I’m saying that however they do, there is the problem of both false positives and false negatives, both of which have serious consequences: where things are identified falsely as being correctly student material, and things where actually they’re AI generated, and the false negatives, I’d argue, could be things where the AI has detected it as being AI generated, but it was actually student generated.
And both of these are problems, and I would argue that if we’re wanting to build reliable education systems, we will never be able to rely on AI detection alone to do that. Because however good the system may be, it will always have some element of false positives and false negatives. And you will never get a hundred percent certainty. And the consequences of the false negatives in particular, can be drastic.
So I would argue that’s a system where, as lecturers, building reliance on the detection is never going to be the right answer. Whatever advances there are in the future, to get a hundred percent certainty both ways on is unrealistic and the negative consequences could be dire. The negative consequences, and again we can go back to this study that we’ve mentioned a lot in the course, it’s one of the motivating studies of the course, at Reading University, it showed that not only were not many of these scripts detected, but for first and second year courses the AI generated scripts outperform students on average. And so therefore the incentives are wrong, that if you don’t get caught, you end up doing better.
So that’s the problem. And the second problem is, if you now don’t use AI and you get labelled as having cheated or used AI, it can have such negative consequences. It’s huge. And then you have to go through appeals, there was this story about somebody who had to actually then under exam situations rewrite something and redo an exam or redo something. And even so, what they created was still identified as coming from AI. And their integrity is just totally questioned, when actually it shouldn’t have been. And you’d expect there to be such cases because of the nature of these systems. They cannot be a hundred percent perfect. You will always get false positives and false negatives.
[00:07:11] Lily: Just to add there in particular with that example, it comes up for particular groups of people. There was an article that came out saying, oh, I saw someone write the word ‘delve’ in an email to me, so I deleted it, assuming it must be AI generated. And then a lot of people from Nigeria then stood up and said, wait a second, that’s a word in our everyday vocabulary. Every time since then, every time I hear the word ‘delve’, my head automatically goes, ‘did AI write it?’, even though it’s people saying it to me in real life, in the real world, and so there’s also just a level of discrimination there.
[00:07:43] David: Exactly. And this is the key point, and this is why it’s so serious and why this would be something where whatever progress gets made here, I would argue that for lecturers relying on this would be the wrong choice. Now, don’t get me wrong, I’m not saying that these things should not be pursued. If you’re a journal and you’re looking at articles, then I think there’s a whole different question about this and processes.
But I would still argue that you need to worry about those false negatives and false positives. And so you need to insert human processes into the loop as well as the AI processes. And however good the AI gets, this isn’t going to solve those issues.
[00:08:22] Lily: Sure, so you feel that these challenges will always be there, of identifying AI generated content.
[00:08:28] David: Broadly, I think this is something to bear in mind that when you are wanting any form of classification and you’re using AI for that classification, there will always be false positives and false negatives, and you should always think about what the consequences of those are for the humans and the individuals involved. So this is just generally, when we are using AI for classification, we need to know that the damage done by the false positives and false negatives is mitigated, and so on. You will not get perfect systems.
[00:09:00] Lily: Great. So should we move to the when?
[00:09:02] David: Yeah, let’s move to the when. I’d like to draw out from the Amazon example, the shopping example.
[00:09:09] Lily: So the “just walk out”. So we discussed this previously in the course, in Amazon, there’s these Amazon Fresh stores where you can walk in, it’s called “just walk out”, it’s a kind of tagline for it, I suppose. And you walk in, you pick things up, you put it in your bag and you just walk out of the shop, and at no point do you have to interact with people at cash points, you just…
[00:09:32] David: Cashiers, yes, yeah.
[00:09:34] Lily: Cashiers is the word, yeah. You just walk out. And then there was a scandal that kind of came out in May or June or relatively recently anyway, in 2024, that actually what’s going on here is the algorithms aren’t in place to actually be able to tell what people are buying and picking up, and I think it was about 70 percent they said of the time it’s by individuals watching you through these cameras and manually identifying your purchase.
[00:10:05] David: And the key point here is that, they built this knowing that to be able to do it, they would need to have huge coding processes, where you’ve got this huge effort to code up and to get those AI systems to learn. And after multiple years of doing this, the AI systems were still not reliable enough. And hence, they were still using human effort to largely do the bulk of the work. And the problem was in many ways that this was a harder problem than they had imagined. And the AI systems weren’t powerful enough.
But that’s just a matter of when. You break this down into a small task, it’s solvable. The problem is the scale of the number of products, and the complexity of that as a big task. You had it in a much smaller shop with a very limited number of products, my guess is it would be solvable right now. Scaling that up is something which is a matter of when it will be possible to scale this up, not if, in my opinion.
Now that “when” might be 20, 50 years down the line, but it’s something where the current technologies are probably sufficient to solve this problem eventually. I’m not saying that we should therefore pursue this until the problem is solved, because I’m actually quite happy that this has been brought out and we’re not pursuing this in that way now.
But I do feel that’s a really good instance of something which is an eminently solvable problem with the current technologies, but which can’t currently be solved. Great. It’s just a question of when. And there are other examples of this.
So let me come back to education on this, in some sense. And there’s a lot of instances of this that I would see in education, where there are problems which, if the right amount of money was thrown at it, you could solve them with the current technologies, but the solutions are not currently there. And most of these are relatively niche, which is why the right amount of money is not getting thrown, it’s not cost effective.
But there are problems, and there are quite a lot of them out there, where although the tools may not currently be able to do them, if you were to use the current tools, and really train them right, and go through the processes, you could create things to be able to give feedback in certain ways, do elements of grading in certain ways, I would argue, that are currently not possible with the tools, but could easily be.
[00:12:49] Lily: Interesting. So these sort of tools where we know that AI will continue to evolve over the course of the next many years, and your kind of claim is that one day these can be possible. It’s a matter of when, not if. Sorry, a matter of when not never.
[00:13:12] David: Yes, exactly. I’m wondering how specific I should get on this, but being able to take a text and, I want to be careful because, other people may claim that’s already possible, so I don’t want to be too specific here. But many different tasks that you could imagine might be possible with AI, I would argue, they could be classified into this type of task. And it would be a really interesting discussion to pick out specific cases that people have and go through them, where, if you put the right experts on it and you put enough resource into actually training the models on it, I believe there are many tasks in education where we could have advances which don’t currently exist with the current technologies. And this is where a lot of ed tech solutions may be coming in and wanting to identify and to find those.
[00:14:12] Lily: That’s really, that’s exciting. So this could be something in 20 years or it could be sooner but it’s…
[00:14:19] David: And what gets developed will be where the money goes, unfortunately. It’s where the effort is put. And actually, if there are things which are of value and they’re not possible in the current systems, it may be worth investigating, is this something which could be done?
It might be in some cases it doesn’t take much effort, in other cases it might take a large amount of effort. The Amazon stores example is a wonderful example where the reason that they went ahead with this is that they knew that this was a solvable problem, but they underestimated exactly what it would take to make it a reality, and it was too big.
And that’s going to be, that’s going to be the case in a number of these instances where that balance of between what is theoretically possible and what can actually be trained in the right ways with the right data, that difference, I’m not sure that people will be able to, just like in the Amazon example, always predict whether or not, how long it will take, what resource level it will need, to produce these valid solutions.
[00:15:25] Lily: So I’m just now scanning through different case studies in my head and I’m wondering, do I put them in the “never” or in the “when”?
[00:15:31] David: This is the thing, and this is where we then get to the next example, which I think is a really interesting one. And for me, the example I have on this is something like Khanmigo. They, once ChatGPT came out, they said, this is it, at last we could get private tutors available to anyone enabling much deeper learning. And so if only we could get and train AI private tutors, we could get improvements in education, which would be fantastic. And they had a substantive advance in what they actually have found, in my opinion, which is to separate out the content piece of understanding what is the answer from the style piece of understanding how should this answer be presented back to the student, in other words, the tutor piece.
And we’ve argued elsewhere that broadly AI at the moment is really good at that style piece and less good at this point at the substance piece. But just recently there have been real advances. Less than a week ago from when we’re recording this, about the 25th of July 2024, there was a Nature article that came out, I believe, it had both a, if you want, a proof based system and a geometry based system, which did pretty well, they got a silver medal on the maths Olympiad resources. And what that means is that it’s doing substance for mathematics.
[00:17:08] Lily: And the Maths Olympiad is this set of questions, eight questions you’re asked, and generally it’s the best…
[00:17:16] David: This is the brightest young mathematicians, sort of high school level mathematicians in the world compete on these really rather hard questions. If you get a gold medal, you are one of the top young mathematicians in the world. If you get a silver medal, you are absolutely exceptional. And already on this. These AI systems were able to, give or take, get silver.
And what was very interesting, there’s a number of details on this which we won’t go into in this episode, but one of the things that I found really interesting about that, was that actually, in one case, the solution the AI system came up with was imaginative and new, and is not one that would have been so easily found by humans. It was not a known sort of solution, the top class mathematicians were looking at that and saying, wow, that’s interesting and new.
So it’s something where the value of AI for the substance part, the actual, in mathematics, the mathematics, rather than just the presentation of mathematics, this is something where there are advances happening right now, which will change the balance of what’s possible in the future.
That based on a certain level of large language model input, you can only get so far. But once you can get these AI models, which can actually do the maths properly, that will change a lot. And it’s not just maths, of course, it’s other subjects this is going to come into. So being able to have really powerful AI systems for the substance, I would argue that’s what’s going to open the door to future advances.
And these could be really exciting and they could be coming sooner rather than later, as has been shown with these advances that DeepMind has had recently, but they need an advance in the technology, I would argue, it’s not just a question of more of the same. So that’s where I would distinguish this from the second category where, the current technology is possibly sufficient, but the complexity of the problem means that solving it may take a long time or it may need to be broken down into lots of little pieces and so on.
Whereas this example coming out of some of these DeepMind algorithms, where they are actually able to do substantive mathematics, will change what is possible by the systems.
[00:19:47] Lily: So exciting. Really exciting to see these breakthroughs and what can be quite soon, relatively.
[00:19:53] David: We don’t know what quite soon might mean. I would argue that this is something where some of those advances are on the cusp and they will have substantive impact, just as ChatGPT had substantive impact once it reached a certain level. And so I think there’s possibilities there emerging.
[00:20:12] Lily: Great. And then what about these unknowns?
[00:20:14] David: My favourite case study for this is diversity in Gemini, I believe it was.
[00:20:21] Lily: Yes, in Google’s kind of AI system to generate images where they were being overly diverse to the point that it didn’t make sense in the context. Such as having over diversity in images generated of the founding fathers of America signing the bill or, of someone generated images of Nazis and you had a lot of diversity in that, in Germany, sorry.
[00:20:50] David: Now don’t get me wrong, what they were trying to do was very good, but it was leading to historical inaccuracies. And this is what’s actually so important, that line between, when it’s just generating images, what it’s generating is not real. But when you’re generating something which is historic, it is real. There is a historic element to it. And so, that balance between the two, I don’t know that we’re ever going to be able to get systems that really deal with this well.
This is a point at which I would argue that we, there are real question marks. I have a lot of admiration for what their team was trying to do, but I’m also rather surprised that they didn’t recognise some of these limitations that are related to this. Because it’s not just that this is a hard problem, it’s not clear to me that this is a solvable problem.
[00:21:49] Lily: I see, this is why you’re saying it’s an unknown. It’s a “will we be able to solve it”?
[00:21:54] David: Yes, exactly. This could be that actually I’m just not imaginative enough and then within the current technologies it could be solved, it’s just a matter of hard work and then it’s category two. Or it could be that there will be advances which mean that we’re able to solve it by understanding the substantive differences between history and not history and actually building that into the underlying models, which is three.
Or it could be that whatever advances that come, we’re never going to be able to make that distinction between fact and fiction, and so actually this is one. And I, in this particular case, I don’t know, and I don’t think anyone knows. I think a lot of us hope that we will be able to do better than we can currently do.
But this is something where I would be very hesitant to take anything on and promise it in this area because I don’t believe, I don’t know that it’s possible. I don’t know what is possible is maybe the correct statement. And I don’t believe anyone really does. And I think that’s fine, recognizing that there are still areas where nobody really knows exactly what is possible.
That’s fine. This is part of what’s exciting. This is where things will be found out in the future. But even that distinction about whether it needs new technologies, whether it can be done in existing technologies, or whether it will never be achievable, is a useful thought exercise to be able to think through.
And I would argue that if you think about that classification, it’s actually a surprisingly useful classification. And I’m really keen to be challenged by people to say, take this specific example, could you classify it? I don’t know that I’d get it right all the time, but I think almost always that discussion of understanding these different categories of things to the future, what could AI do in the future?
It’s possible that that classification into these four types could be a useful discussion for non experts, for lecturers wanting to use AI in certain ways, to have with experts. If you’ve got an idea of how you want to use AI, which isn’t working within the current tools, talking to an AI expert to understand, could you help me understand where might this problem lie?
That’s the sort of thing which I think would be really valuable and really useful. And I think it’s surprising how often I find I can stick a stake in the ground and put my opinion on where something would lie on this. I would argue that, let’s say, the idea of having personal tutors I would argue that’s firmly in that third category for me. It needs these content based advances. Without those, I’m not going to be happy with those personal tutors, the AI based personal tutors. But with the sort of advances that I think are possible there, I think good AI personal tutors are possible. I’d stick a stake in the fact that’s a third category one for me.
And I could go through in other cases in education of what I think would be possible. I won’t always get it right, but I feel that’s what we should be doing more. And that’s what we should be helping people to communicate more because it’s not easy for a non specialist. It’s not easy for specialists to know where things lie along that sort of spectrum and different people will disagree.
But it is, I think, really useful for debate and discussion. I can articulate, again, I’ll use the case of the personal tutor. I can articulate the reason I don’t think the current technologies are there, and it’s not just a matter of when, is because even if you do separate out and have multiple layers and add the complexity, unless you have that reliability of the substance, there are going to be bits that it might, you might get something which would work really well, but it wouldn’t be as trustworthy as I’d want. Whereas the advances that are coming out related to mathematics specifically, I would argue that would mean that for a mathematics personal tutor in the future, I would imagine we could get something reliable enough. So that would be where I would put that differentiation. And I think this is consistent with what I’ve also been reading others who I think the advance of doing this would be thinking as well.
[00:26:33] Lily: Really interesting. So there’s these different ways, then that we’ve discussed of how AI is likely to evolve in the future, but then what can lecturers do about this?
[00:26:42] David: I would argue that the thing which is really useful to think of now, and the reason I think this is a good way to finish the series, is that if you think about that fact, let’s take, there’s two things I put a stake in the ground on explicitly here.
One is that detection will never be something you should rely on. And I think that’s something which if as lecturers, we take that and people can argue that differently, but as lecturers we agree that is a position to take, well great, we can build education systems around that. And we can know that those education systems will probably still be valid in the future as they are now.
Similarly, if we think that these personal tutor will be available in the future, we can try to think, okay, we don’t have access to that right now, but what do we design in terms of our education systems that would be robust to that if that were available in the future? And where the value of the education, the human education we give, would still add value on top of that.
So if and when that advance hits us, we’re ready for it. And this is where I think, I’m not worried about people being ready for when the detection is perfect, because it’s not going to be perfect. And so I’d like people to always be designing with that in mind. But I would like people to be considering what sort of education could they be giving if they could be essentially getting to the point where the AI could be providing the equivalent of private tutor sort of support to individual students.
That would be really interesting. And then designing the education to complement what a AI, private tutor support could give on top. And that might mean designing more for group work for human interaction, because that’s the sort of thing that the AI private tutor can’t do. So you’re building in elements which are automatically, even if other elements of the education, now students are able to learn that through their AI private tutors. The education itself offers a better education because you have the group elements, because you have human interaction built in. And those are the sort of things which I think would be really valuable for lecturers to be thinking through as they design for now, but also for the future.
[00:29:15] Lily: Excellent. Thank you very much, David. It’s been a pleasure as always, and really enjoyable to do this course with you.
[00:29:21] David: Yeah, I really enjoyed this and thank you for making this happen. It’s been a pleasure and I hope people find it useful.
[00:29:29] Lily: Yes, yeah, and I look forward to reading feedback and looking at things on the forum rooms as well.
[00:29:35] David: Thank you.
[00:29:36] Lily: Thank you.